THE PROJECT
Using contemporary mathematical methods, our aim is to enhance assessment of individual cardiopulmonary exercise function. To achieve our mission, we require exercise test data from laboratories around the world to create a reliable cardiopulmonary function test interpreter.Â
Experts contributing to Oxynet will become masters of the system, bringing forth a collective intelligence that could not be done alone. Simply put, Oxynet will enable enhancement of our shared knowledge in the area of cardiopulmonary function testing, allowing participants to gain back even more than they give.
A CROWD-SOURCING WEB APPLICATION TRAINING NEW INTERPRETERS OF CARDIOPULMONARY EXERCISING TEST DATA
Ventilatory thresholds and maximal oxygen consumption are important physiological parameters we can obtain from cardiopulmonary exercise testing (CPET). These markers give insight into aspects of an individual's physical fitness, health and performance potential. By visually inspecting CPET results, patterns of ventilatory data variations offer insight into their likely physiological underpinnings. Machine learning algorithms have the potential to approximate human-level analysis of CPET data. We believe that the spread of artificial intelligent technologies in CPET data interpretation is currently hindered by the limited data available to the communities of developers. Therefore, Oxynet was formed as a web-based application to facilitate the collection of large CPET datasets that will be used to train and test new machine learning algorithms.
HOW IT WORKS
1. Experts upload their CPET files by means of the Oxynet web-app
2. Data are collected on a server and analyzed by a neural network
3. In the future, the neural network will be used to interpret unlabelled CPET data

DATA TREATMENT
1. Uploaded files can be deleted any time by the user
2. Users are responsible for the data provided. We strongly encourage users to:
2.0Â Use old databases retrospectivelyÂ
2.1Â Anonymize the data before uploading
2.2 Use data that has been collected with approval of an ethical committee
2.3 Use data that has been collected with informed consent from the subjects/participants/patients
2.4Â Cite the research work or publication where the data have been used
GET INVOLVED
Do you have data that you can share with us?
Read: How to Contribute to Oxynet With Data
Can Oxynet be of help in your ongoing and future projects?
Let us know!
CONTACT US
Join the discussion on Slack
Follow the project on Research Gate
Trentino Sviluppo
Via Fortunato Zeni 8, 38068, Rovereto, Trento
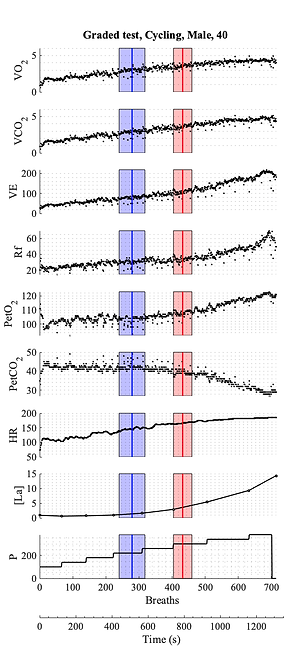
IN MORE DETAILS
Why we intend to leverage expertise with computers
Cardiopulmonary exercising test (CPET) is used to assess the responses of an individual's cardiovascular and ventilatory systems to a known increasing working load (Wasserman, Hansen, Sue, Stringer, & Whipp, 2005). The test is usually conducted on a cycle ergometer (cycling) or on a treadmill (running or XC-skiing). The power output at the cycle ergometer or the speed of the treadmill belt are progressively increased at a constant rate (CPET ramp test) or every 3 or 4 minutes (CPET graded test) until participant's volitional exhaustion (Bentley, Newell, & Bishop, 2007). During the test, a metabolimeter is used to measure the flow and the O2 and CO2 concentration of the gas exchanged at the airways, that are tightly coupled with cardiac output, pulmonary blood circulation and peripheral O2 extraction (Jones & Poole, 2005).
Typically, the following variables are collected during a CPET (see Fig. above): O2 uptake (V̇O2), exhaled CO2 (V̇CO2), ventilation (VE), respiratory frequency (Rf), end tidal O2 (PetO) and CO2 (PetCO), heart rate (HR) and working load. To these cardio-respiratory variables, some prefer to associate a metabolic variable, like the blood lactate concentration ([La]), and to use lactate thresholds (Faude, Kindermann, & Meyer, 2009)along with ventilatory thresholds. However, due to the metabolic-cardiovascular coupling, all these thresholds are closely related and appears to be associated to the same physiological events (Rossiter, 2010).
During a CPET, breakpoints in the ventilatory variables identify important indexes of athlete's exercise capacity and can also be used to assess the effectiveness of a training program on the athletic performance:
​
VT1, the first ventilatory threshold identifies the upper limit to the moderate intensity domain, and it corresponds to: 1) the first disproportionate increase in VE; 2) an increase in VE/V̇O2 with no increase in VE/V̇O2and 3) an increase in PetO with no consequent fall in PetCO.
​
VT2, the second ventilatory threshold identifies the upper limit to the heavy intensity domain, and it corresponds to: 1) the second disproportionate increase in VE; and 2) the first systematic decrease in PetCO and 3) the first systematic increase in VE/V̇CO2.
​
V̇O2MAX, the maximal O2 consumption, identifies the upper limit to the severe intensity domain and it corresponds to the submittal plateau of V̇O2 reached at the end of the test.
​
Usually, ventilatory thresholds are expressed in terms of a time from the beginning of the test, or by means of the associated V̇O2, HR or intensity. CPET is worldwide known procedure among sport scientists and there is a large literature on how to design and conduct a valid and informative CPET. For example, Wasserman et al. (Wasserman et al., 2005) dedicated an entire book to CPET design and interpretation and Robergs et al. (Robergs, Dwyer, & Astorino, 2010) recommended the methods currently adopted for correct data processing. CPET data interpretation requires expertise and the concomitant visual inspection from multiple reviewers (Prud’Homme et al., 1984). Visual inspection is therefore expensive in terms of time and energy and is affected by intra- and inter-evaluator variability (Gladden, Yates, Stremel, & Stamford, 1985). Particularly, inter-evaluator reliability has a direct effect on the reliability of the visual method (Prud’Homme et al., 1984).
​
With the ultimate goal of creating an objective tool for CPET data interpretation, computerised methods have been developed for automated ventilatory threshold detection (Ekkekakis, Lind, Hall, & Petruzzello, 2008) and V̇O2MAX estimation. Currently, the reliability of automated methods is hindered by to main issues: 1) they handle only one ventilatory variable at time and 2) the typical noise underlying ventilatory signals (Keir, Murias, Paterson, & Kowalchuk, 2014) impairs their break-point detection ability. Artificial intelligence algorithms, such as neural networks, can be used for CPET data interpretation (Baralis et al., 2013; Hearn et al., 2018) and, most importantly, their performance can improve if new labelled data is provided for supervised training (Längkvist, Karlsson, & Loutfi, 2014; Zignoli et al., 2019). In addition, artificial intelligence algorithms are less sensitive to the signal-to-noise ratio and they can easily handle multiple variables at the time, hence they can be used to individuate complex pattern of variations in ventilatory variables.
​
In general, we can define artificial intelligence in CPET interpretation as the use of algorithms and softwares to approximate human cognition in the analysis of CPET data. As one of the biggest limitation to the development of new artificial intelligence algorithms is data availability, our aim is to develop a crowd sourcing web-application to collect data and train and test new artificial intelligence algorithms for CPET data interpretation. The development of new artificial intelligence algorithms will hopefully lead to a new generation of reliable CPET interpreters, that could support experts during visual-inspection analyses.
​
References
Baralis, E., Cerquitelli, T., Chiusano, S., D’elia, V., Molinari, R., & Susta, D. (2013). Early prediction of the highest workload in incremental cardiopulmonary tests. ACM Transactions on Intelligent Systems and Technology, 4(4), 1–20. https://doi.org/10.1145/2508037.2508051
Bentley, D. J., Newell, J., & Bishop, D. (2007). Incremental Exercise Test Design and Analysis: Implications for Performance Diagnostics in Endurance Athletes. Sports Medicine, 37(7), 575–586. https://doi.org/10.2165/00007256-200737070-00002
Ekkekakis, P., Lind, E., Hall, E. E., & Petruzzello, S. J. (2008). Do regression-based computer algorithms for determining the ventilatory threshold agree? Journal of Sports Sciences, 26(9), 967–976. https://doi.org/10.1080/02640410801910269
Faude, O., Kindermann, W., & Meyer, T. (2009). Lactate Threshold Concepts: How Valid are They? Sports Medicine, 39(6), 469–490. https://doi.org/10.2165/00007256-200939060-00003
Gladden, L. B., Yates, J. W., Stremel, R. W., & Stamford, B. A. (1985). Gas exchange and lactate anaerobic thresholds: inter- and intraevaluator agreement. Journal of Applied Physiology, 58(6), 2082–2089. https://doi.org/10.1152/jappl.1985.58.6.2082
Hearn, J., Ross, H. J., Mueller, B., Fan, C.-P., Crowdy, E., Duhamel, J., … Manlhiot, C. (2018). Neural Networks for Prognostication of Patients With Heart Failure: Improving Performance Through the Incorporation of Breath-by-Breath Data From Cardiopulmonary Exercise Testing. Circulation: Heart Failure, 11(8), e005193.
Jones, A. M., & Poole, D. C. (2005). Oxygen uptake dynamics: from muscle to mouth–an introduction to the symposium. Medicine and Science in Sports and Exercise, 37(9), 1542–1550.
Keir, D. A., Murias, J. M., Paterson, D. H., & Kowalchuk, J. M. (2014). Breath-by-breath pulmonary O 2uptake kinetics: effect of data processing on confidence in estimating model parameters: Breath-by-breath pulmonary O 2uptake kinetics. Experimental Physiology, 99(11), 1511–1522. https://doi.org/10.1113/expphysiol.2014.080812
Längkvist, M., Karlsson, L., & Loutfi, A. (2014). A review of unsupervised feature learning and deep learning for time-series modeling. Pattern Recognition Letters, 42, 11–24. https://doi.org/10.1016/j.patrec.2014.01.008
Prud’Homme, D., Bouchard, C., Leblance, C., Landry, F., Lortie, G., & Boulay, M. R. (1984). Reliability of assessments of ventilatory thresholds. Journal of Sports Sciences, 2(1), 13–24. https://doi.org/10.1080/02640418408729692
Robergs, R. A., Dwyer, D., & Astorino, T. (2010). Recommendations for improved data processing from expired gas analysis indirect calorimetry. Sports Medicine,40(2), 95–111.
Rossiter, H. B. (2010). Exercise: Kinetic Considerations for Gas Exchange. In R. Terjung (Ed.), Comprehensive Physiology. https://doi.org/10.1002/cphy.c090010
Wasserman, K., Hansen, J. E., Sue, D. Y., Stringer, W. W., & Whipp, B. J. (2005). Principles of exercise testing and interpretation: including pathophysiology and clinical applications. Lippincott Williams & Wilkins Philadelphia.
Zignoli, A., Fornasiero, A., Bertolazzi, E., Pellegrini, B., Schena, F., Biral, F., & Laursen, P. B. (2019). State-of-the art concepts and future directions in modelling oxygen consumption and lactate concentration in cycling exercise. Sport Sciences for Health, 1–16.